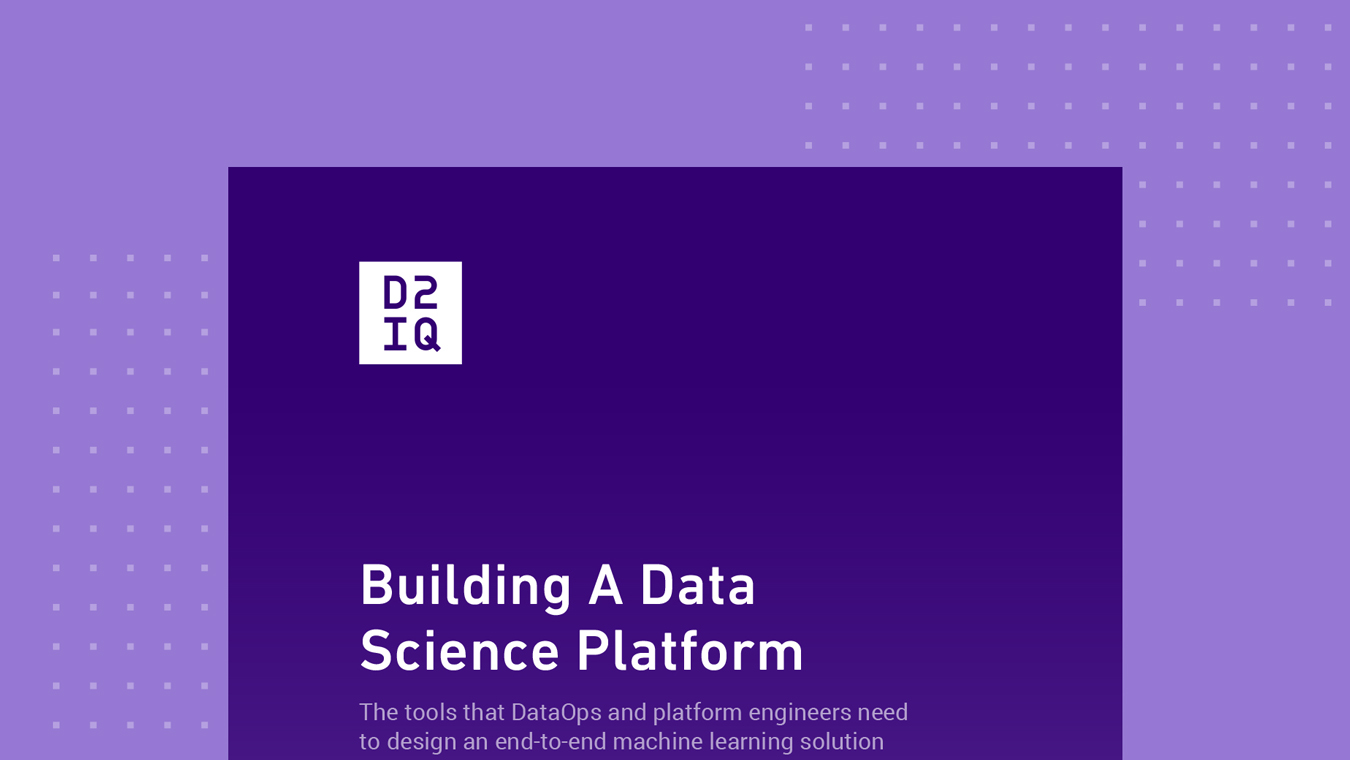
Design & Build an End-to-End Data Science Platform
Learn best practices and tools required to design and build an end-to-end machine learning platform.
In this helpful guide, you will learn:
- How to adopt automated workflows and open source processing tools to save up to 60% of your time.
- How moving to fully distributed environments (vs local environments) can help you reduce friction and increase agility when managing and training models.
- The importance of collaboration across Data Engineers, DataOps, and Data Scientists to ensure the solution is easy to provision and manage day-to-day.